Can AI Think Like Humans? New ‘Machine Memory’ Framework
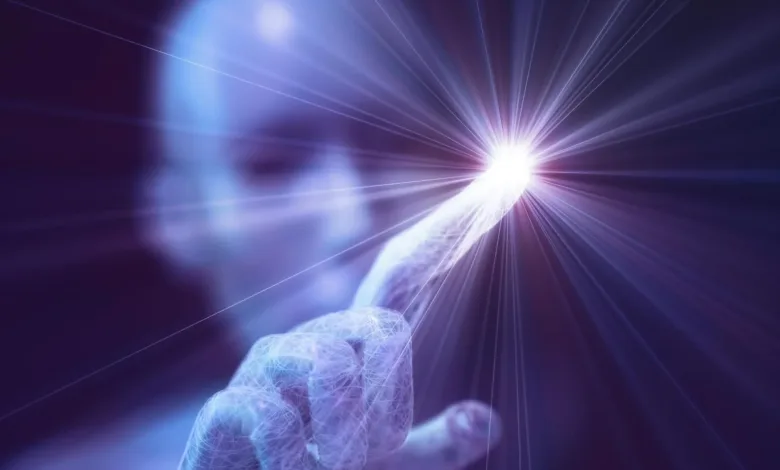
In a groundbreaking development, researchers have introduced Machine Memory Intelligence—a new AI framework inspired by the workings of human memory. This brain-like model could pave the way for smarter, more adaptive machines that learn continuously, reason more effectively, and use energy efficiently. The M2I framework, detailed in a recent paper published in the journal Engineering, offers an innovative response to the limitations of current large-scale AI models like ChatGPT.
The Limitations of Today’s AI Models
Modern AI systems, while powerful, face serious challenges. They require immense data and computing power, struggle with reasoning, and are prone to forgetting previously learned information when updated with new data—a problem known as catastrophic forgetting. The research points out that these issues stem from the current structure and training methods of artificial neural networks, which rely heavily on static, data-driven learning.
Introducing the M2I Framework
This machine memory supports dynamic updates, association-based access, and fuzzy logic operations. The M2I framework brings together three core modules: representation, learning, and reasoning, all interacting in two continuous feedback loops.
Read: AI Takes the Spotlight in MediaTek Dimensity 9400+
Key Areas of Focus
The framework is designed around four major areas of cognitive function, each modeled on human brain processes:
1. Neural Mechanisms of Machine Memory
This area explores how the brain’s pre-configured neural systems and plasticity contribute to intelligence. By studying these mechanisms, the M2I framework aims to replicate similar patterns in AI, enabling machines to adjust and grow intelligently over time.
2. Associative Representation
Human memory excels at linking abstract and concrete concepts and forming spatiotemporal connections. M2I mirrors this by encoding and retrieving data through similar associative methods, allowing machines to organize and access knowledge more naturally and flexibly.
3. Continual Learning
To overcome the hurdle of forgetting, the framework supports continual learning—even under limited power conditions. However This allows AI systems to integrate new knowledge without overwriting what they already know, a crucial step toward long-term adaptability.
4. Collaborative Reasoning
The M2I framework blends intuitive and logical reasoning, seeking to make AI systems not only more effective but also more interpretable. This dual-process reasoning approach could improve decision-making and performance across a wide range of applications.
Shaping the Future of Smarter AI
By aligning machine intelligence with human cognitive principles, the M2I framework could revolutionize the future of AI. This model promises to deliver systems that are not only smarter and faster but also more energy-efficient and context-aware. While more research is needed to bring these ideas fully to life, the potential is immense.
In conclusion this brain-inspired shift marks a bold step toward developing truly intelligent machines—ones that can learn, reason, and adapt just like us.
Follow us on Google News, Instagram, YouTube, Facebook,Whats App, and TikTok for latest updates